Five Essential AI Pillars Shaping Innovation, Growth and Adoption
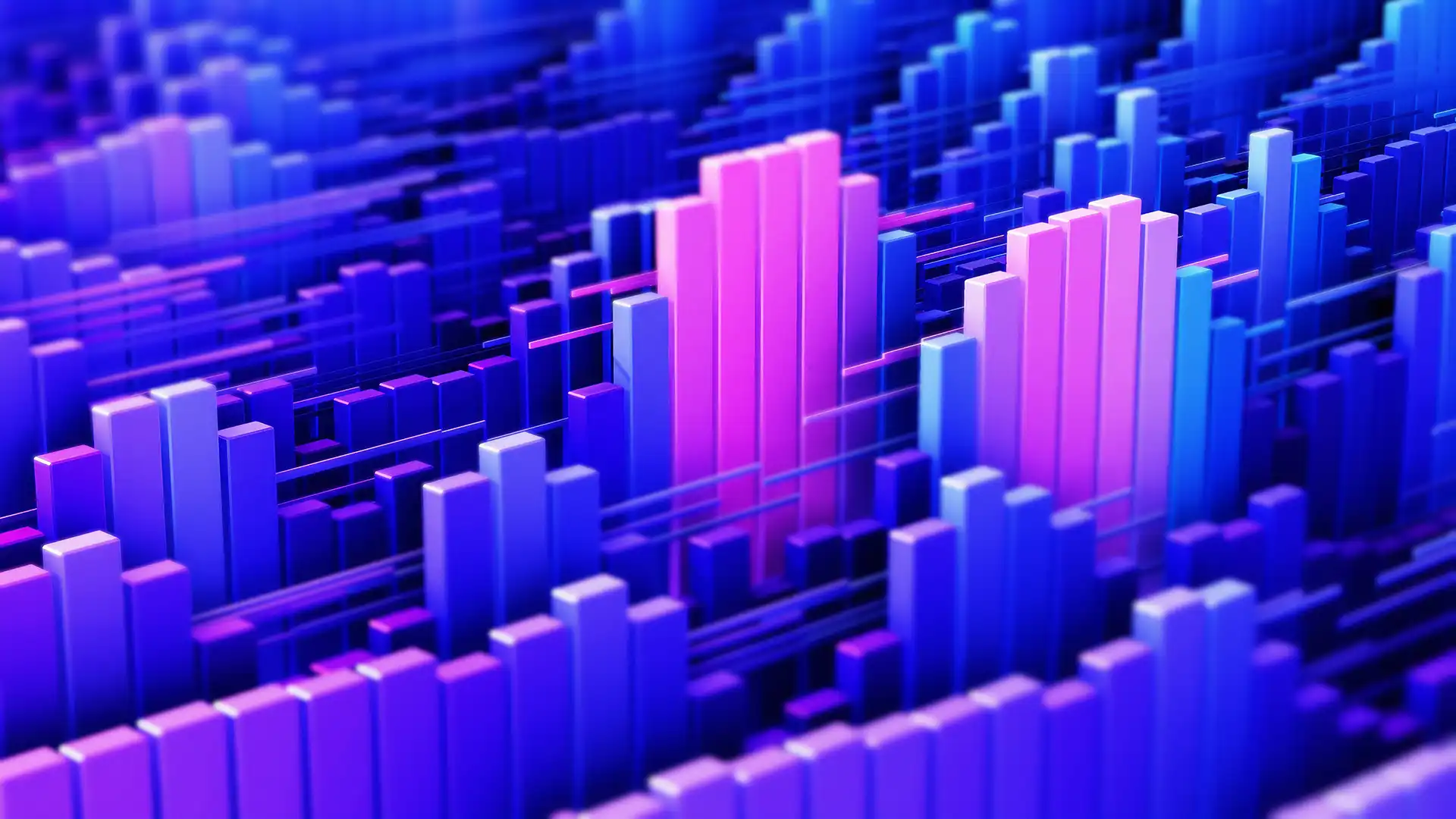
AI continues to deliver and build momentum on being the next innovation driver for enterprises, transforming industrial efficiency and spurring global regulatory bodies to craft new privacy, transparency and cybersecurity legislation.
AI broadly relies on five pillars: 1) Computing 2) Models 3) Data Sets 4) Enterprise adoption and 5) Governance and Regulation. We’ve looked at some of the core developments across 2024 around these five pillars to establish a picture of what enterprises can expect from AI this year. From new breakthroughs in AI chip development to fresh applications in banking, insurance, manufacturing and more, 2025 will set the stage for a more interconnected AI ecosystem – one that offers much greater scalability, while also incorporating much-needed regulatory oversight.
1) Computing pillar – The Optimization Revolution in AI Hardware
Major chip manufacturers including Nvidia, Broadcom, AMD and Intel have been racing to capture the AI hardware market. In part, this is due to the massive computing power that AI consumes, especially when it comes to training LLMs and scaling workloads. Over the past few years, we’ve seen manufacturers come out with new chip architectures and GPUs that are optimized toward AI tasks, giving users better performance per watt and significantly lowered latency for enterprise AI applications. This year, we’re likely to see more specialised systems, that combine GPUs, TCUs and CPUs, to streamline workloads at different stages of the AI pipeline. These will also have an impact on how organizations are able to deploy agentic AI, especially when using multi-agent frameworks at scale.
Cloud computing is also a key priority for chip manufacturers. Nvidia’s DGX Cloud in conjunction with major cloud provider partnerships, for example, is making it easier for organizations to access large clusters of the latest AI hardware to support hyper scaled enterprise processes. These hardware advancements have made it easier for latency-sensitive AI applications to maintain performance, and for cloud providers to build more elastic scalability into their solutions.
At the same time, the not-so-significant power consumption involved in running large AI models has placed new demands on cooling systems and energy-efficient chip designs. Part of the solution has been to use smaller process nodes to minimize power leakage and increase transistor density, so that more can effectively be achieved with a smaller carbon footprint. But as AI models continue to grow in scope and power demand over 2025, chip makers will likely intensify their focus on power management and immersion cooling systems, while cloud computing providers will offer more distributed infrastructure costs to amortize environmental impact and costs over several user groups.
2) Models – AI Matures from Generative to Agentic Models
Over the past year, we’ve seen AI product companies move toward greater product maturity, particularly in the case of enterprise applications. Overall, there’s been a general push toward propelling forward generative AI capabilities and improving AI scalability, while customising solutions to specific verticals. Microsoft’s Azure Open AI, Google’s Vertex, and AWS have all evolved their products to help enterprises train LLMs and multimodal AI systems for specific functions, including process automation, advanced analytics, and content creation. This means that enterprise platforms now contain more robust APIs and interfaces that offer a more seamless user experience, while simplifying integration with legacy systems
Moreover, edge computing and AI platforms are finally achieving seamless integration, making way for agentic AI systems. These systems use multiple real-time data feeds to enable a much more granular degree of autonomous system optimization and process monitoring with fewer human interventions. For example, agentic AI is poised to take over end-to-end workflows in enterprise customer service, IT service management, supply chain logistics, and even industrial automation processes.
Niche applications, hyper-personalized AI models, and possibly AI-powered quantum computing are all primed for exploration this year, as there are more sustainable AI operations and new advances in scalability.
3) Enterprise Data Accessibility: Rise of Synthetic Data
High quality data is important for model’s accuracy. Organizations focussed on exploring AI should review data readiness including Data culture, data governance, data ownership for their enterprise data, in addition look at combination of data procurement and synthetic data to ensure Models are effective and accurate. Synthetic data would drive model accuracy and address privacy and copyright concerns.
4) The Expanding AI Footprint in The Enterprise Landscape
In terms of practical adoption, AI has seen significant growth within manufacturing, especially within predictive maintenance. For process driven enterprises, adaptive process /manufacturing which dynamically adjusts / tweaks the parameters on the fly to drive high quality and productivity will be significant differentiator. Intelligent systems to drive predictive maintenance and auto-corrections will be on the rise. Enterprises like Siemens and GE have already deployed AI to analyse sensor data from their industrial equipment, using advanced adaptive learning frameworks to predict machine failures and streamline maintenance schedules with greater degrees of precision, further reducing expensive downtime.
Digital twin technology has matured in tandem with generative AI to drive real-time process simulations and optimize plant design, with fewer and fewer prototyping cycles. For major industrial manufacturing sites, computer vision systems backed by AI analysis are essential to quality control, using new neural network advances to improve defect detection rates and identify production anomalies even in dynamic production environments.
This year, manufacturers will likely entrench autonomous systems, driven by AI, to enhance production efficiency. We’ll see more deployment of edge and agentic AI tools, trained on federated learning frameworks and a larger role for AI in manufacturing for increased efficiency, when it comes to real-time decision making on factory floors.
For non-industrial enterprises, personalized customer experiences are going to see rapid growth as AI tools are linked to real-time data feeds and the AI data architecture continues to mature. Natural language processing (NLP) bots have entirely transformed help desk interactions and can now handle increasingly complex customer queries, taking the load off human agents. Similarly, insurance companies are likely to deepen their relationship with AI over the course of 2025, deploying vertical-specific tools to integrate both structured and unstructured data sources into claims underwriting processes. This enables them to accelerate and refine risk assessments, premium calculations, and scale operations without needing to scale their work force.
The AI revolution in 2025, will also impact enterprise AI adoption, particularly in fraud detection and process automation functions within financial institutions. Graph neural networks and time-series models, for instance, are being used alongside massive customer behaviour data sets to detect transactional fraud in real-time. Furthermore, compliance systems use AI for automated auditing and regulatory monitoring, employing anomaly detection to flag inconsistencies and potential violations. Through the year, the technical sophistication of AI in financial services is likely to grow with more explainable AI protocols positioned to address a growing regulatory demand for transparency in key AI decision-making areas like claims assessment, portfolio management, catastrophe forecasting, and credit risk.
5) Governance and regulation – Balancing Innovation & Oversight in AI Regulation
Around the globe, governments are formulating new legislation to regulate AI usage, with data privacy concerns, explainability requirements, and security risks at the forefront. In the EU, the AI Act is attempting to stratify AI use into risk brackets, driving new compliance norms for high-risk applications in finance, healthcare, and insurance. Similarly, in the US, the National Institute for Standards and Technology has recently released an AI risk assessment framework that helps enterprises establish greater accountability via audit trails, and cross-border data handling protocols.
Many governments are also pushing for greater AI innovation, and a renewed focus on sustainable and more secure AI ecosystems. In India and Singapore, education initiatives are leveraging AI to expand the skilled workforce pool, while programs like the United Kingdom’s AI Research Hubs are highlighting the need for greater public-private collaborations on AI technology, especially when used within key energy and logistical infrastructure projects.
Propelled by OECD and UN mandates in 2025, AI legislation is expected to achieve more standardization on a global scale and improve data interoperability across international borders. Overall, from a regulatory standpoint, we’re seeing a delicate balance between fostering an AI innovation and growth culture and limiting the potential risk of a still evolving technology.
About Author:
Chandrashekar Surbhat, Senior Vice President – AI & Digital at SLK Software, leads the company’s AI and digital transformation arm, focusing on accelerating business outcomes and innovation. With over 25 years of experience, including a significant tenure at Wipro, he has driven digital and AI initiatives, managed billion-dollar P&Ls, and successfully transformed organizations. Chandra holds a Bachelor of Engineering from Bangalore University and certifications from IIM Bangalore and Stanford, with expertise in AI, cloud, and digital experience. His expertise lies in leveraging strategic alliances and cutting-edge technologies to drive AI-powered digital transformation for clients.