Generative AI Explained!
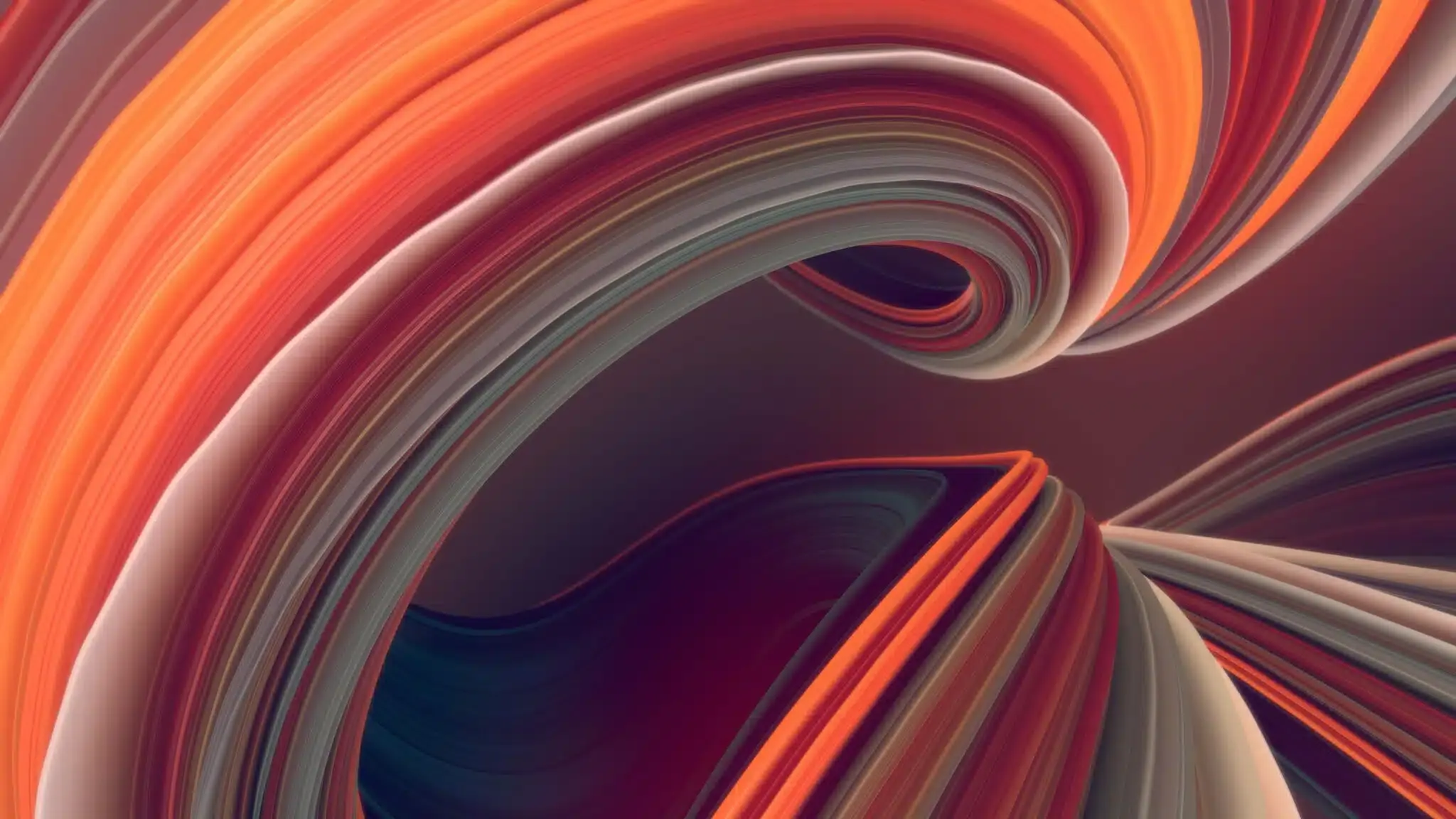
All the buzz around generative AI has made it part of everyday conversations. However, just because a technology is nearly ubiquitous doesn’t make it understandable. So, in the middle of all the technical terms and models surrounding the generative AI conversation, we thought we’d take another angle to it. And so, we asked ourselves, “What if you had to explain this to a 15-year-old?” And here is what followed:
A 15-year-old gets introduced to generative AI
As a software engineer, it’s Mindy’s job to stay up to date on technologies in her field. Learning about new tech is her passion, and some of that excitement has passed on to her 15-year-old son Alex. He’s always curious about new tech and excited to dig deeper into how things work. The latest thing that has caught his attention is a demo he saw at school. Alex waited for his mother to come in from work, and as soon as Mindy got home, he bubbled over.
Alex: Mom, today I witnessed something incredible! My music teacher created brand new music in just two minutes with some sort of application. How is that even possible?
Mindy: Hello to you too, Alex! To answer your question, it must have been generative AI. Have you heard of it?
Alex: No, what is that?
Mindy: Well, it’s a type of artificial intelligence that can create new content, like images or text, based on patterns it has learned from existing data.
Alex: That sounds amazing! How does it work?
Mindy: Generative AI uses a type of neural network called a generative adversarial network (GAN) or a variational autoencoder (VAE) to analyze and learn patterns from a large dataset of existing content. Once the model has learned these patterns, it can generate new content similar to the original data but with unique variations. It has three main parts:
- Generator – The generator creates new content based on the patterns it has learned
- Discriminator – The discriminator checks the generated content to see whether it’s real or fake
- Training data – The training data is used to teach the generator and the discriminator
Mindy: Sure. I don’t know if you remember, but when you were younger, you only knew of Tesla as the make of every car. So, any car you saw, you referred to it as a Tesla. So, here ‘you’ are the generator. Slowly your father taught you about the various car brands and models. He was feeding you training data. Other than giving you more information about different types of cars, your father also corrected you when you made a mistake in identifying a car’s make. That is the discriminator. So, using these three elements is one way of building intelligence.
Alex: That’s really interesting! Can you give me an example of how such a model will work?
Mindy: Sure. Let’s say we train a GAN on a large dataset of paintings by famous artists. The GAN will analyze the paintings and learn patterns such as each artist’s style, color palette, and brush strokes. Once it has learned these patterns, it can generate new paintings that look like they were created by one of those artists but with their own unique elements.
Generative AI has many potential applications, such as creating new designs, music, and drug compounds. For example, a generative AI model could be trained on a dataset of molecules and then used to generate new drug compounds that have never been discovered.
Alex: Wow, that sounds powerful! But are there any limitations to generative AI?
Mindy: Yes, there are some limitations. For example, generative AI is only as good as the data it’s trained on, and it can sometimes create biased or inaccurate content. And for all its capabilities, Generative AI can’t have original creative thoughts like humans. Instead, it can only synthesize patterns and data to create something new.
Alex: I see. Thanks for explaining generative AI to me, Mom!
Fun Fact! Even this blog is generated using Generative AI
To get into the shoes of a 15-year-old, we prompted ChatGPT and created this blog based on the responses. Of course, to make to better we did work some human magic on it!