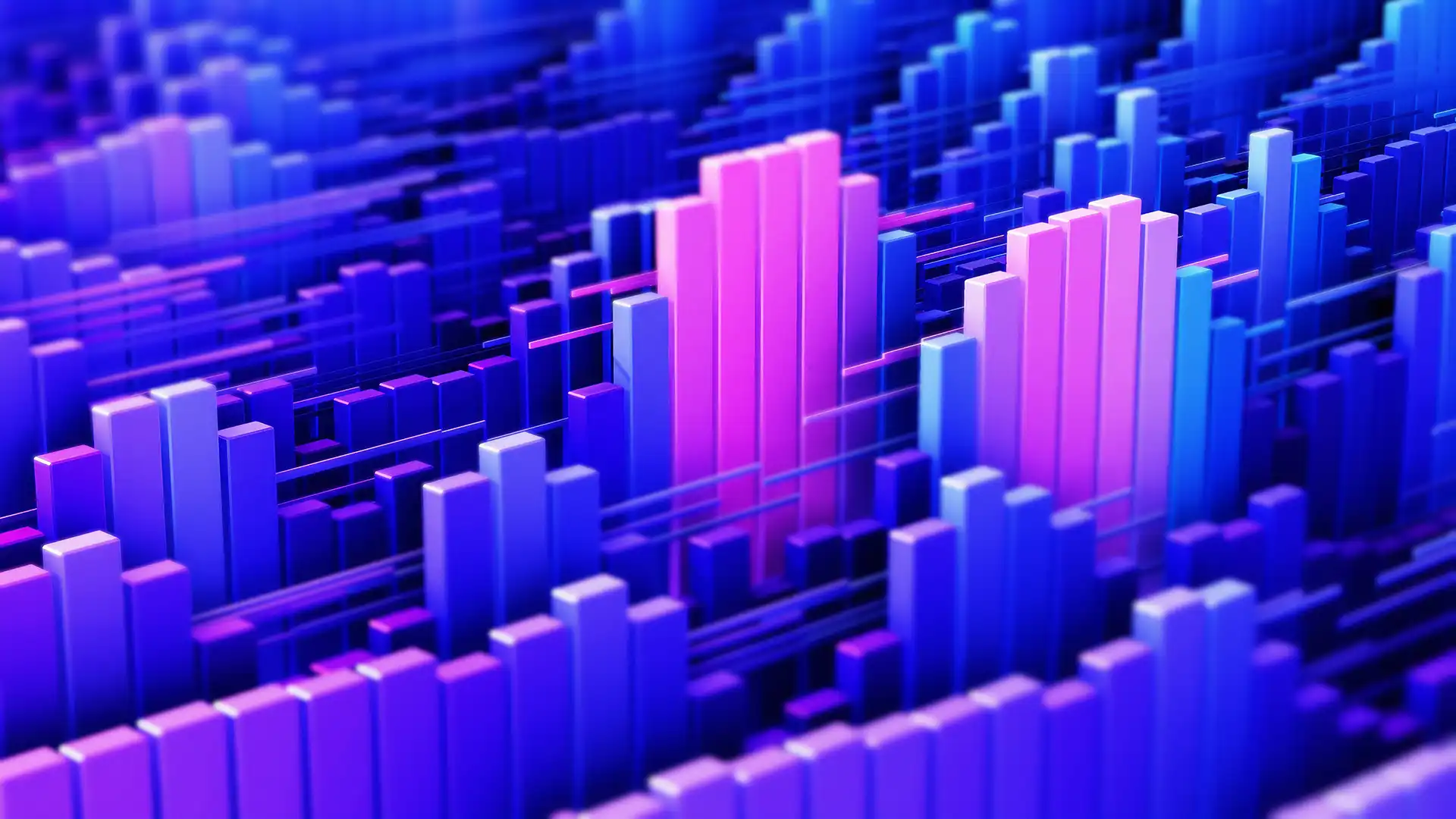
Five Essential AI Pillars Shaping Innovation, Growth and Adoption
Discover the five key AI pillars driving innovation, fostering growth, and accelerating adoption across industries.
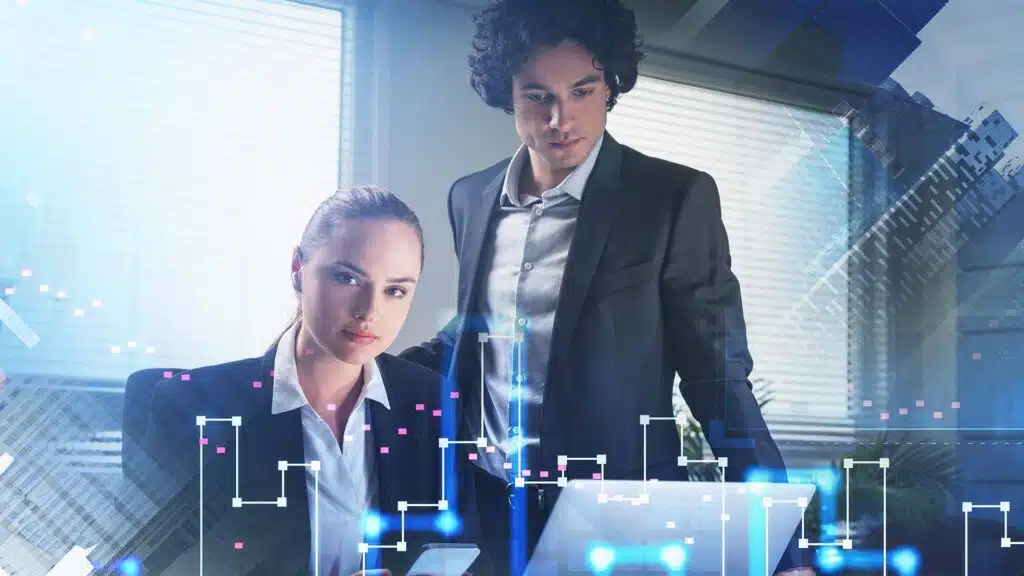
Beyond the Buzz: Making Generative AI Work for Your Business
Discover six key factors for integrating Gen AI into your business strategy. Learn how to embrace the future of AI technology.
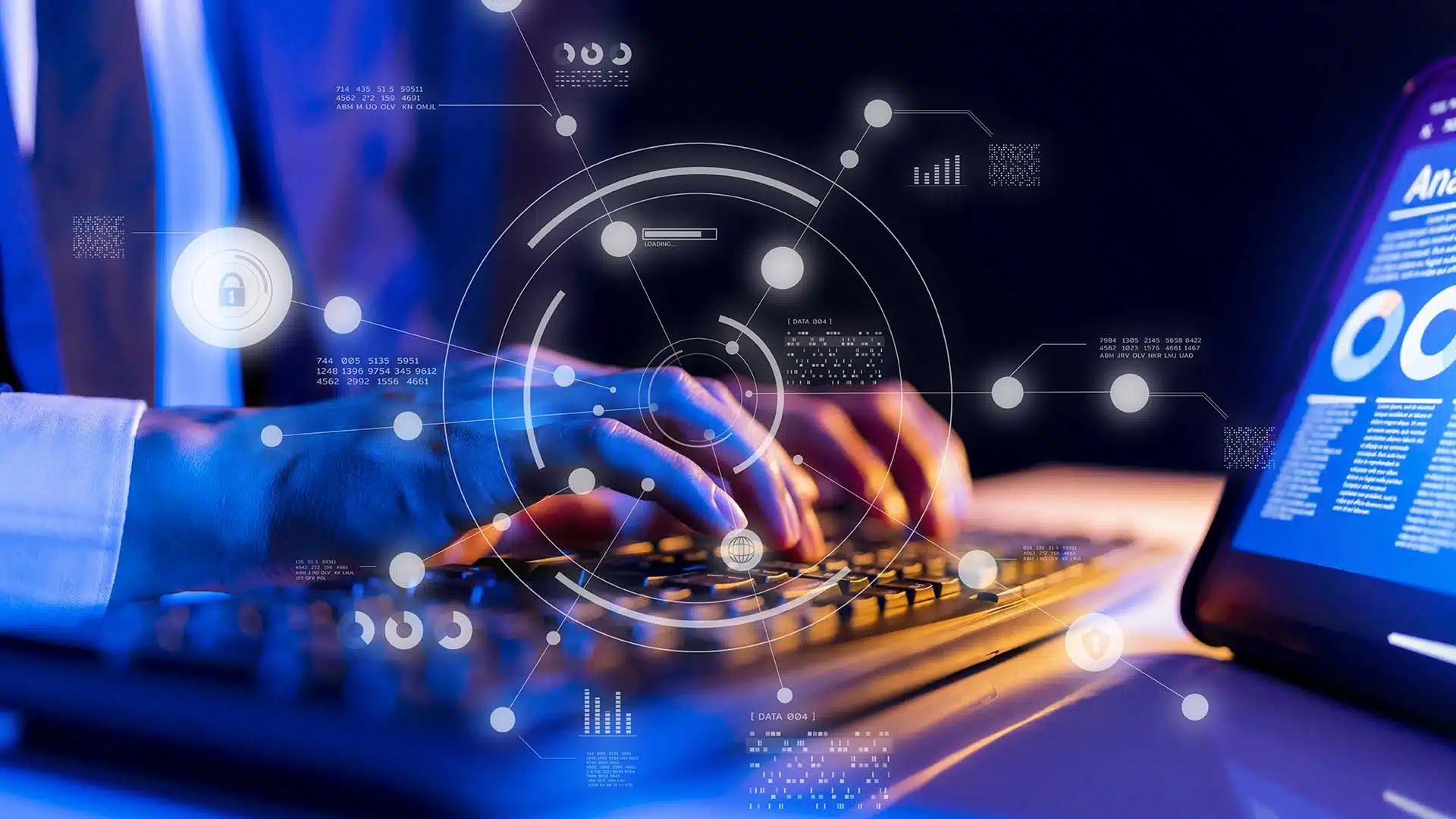
GenAI Unleashed: Supercharging Workplace Efficiency and Creativity
Discover the power of GenAI, the ultimate solution for boosting workplace efficiency and unleashing creativity.
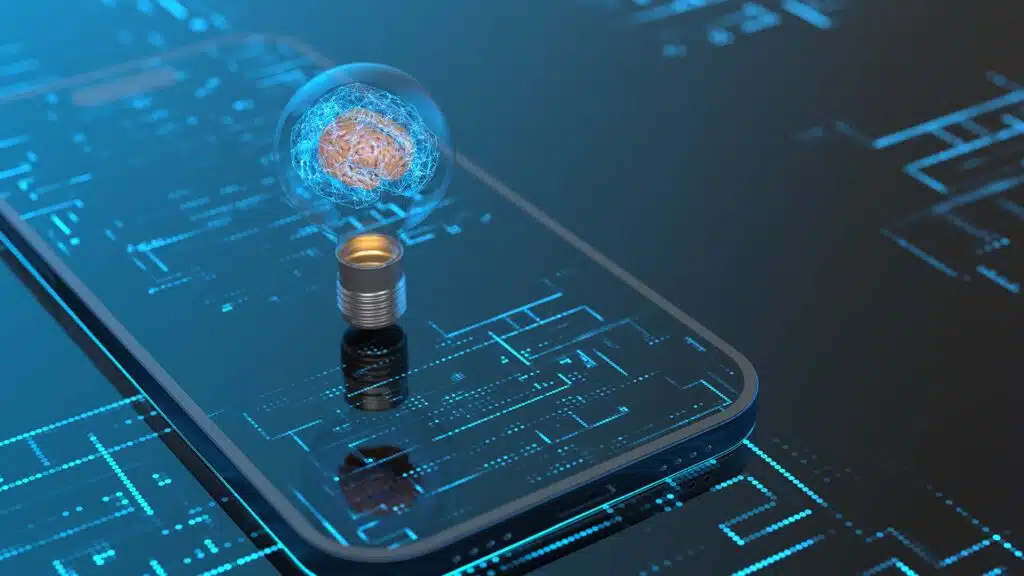
Navigating GenAI's Ethical Maze of Innovation and Responsibility
In this Blog, Explore the intersection of innovation and responsibility in GenAI's ethical maze of navigation.
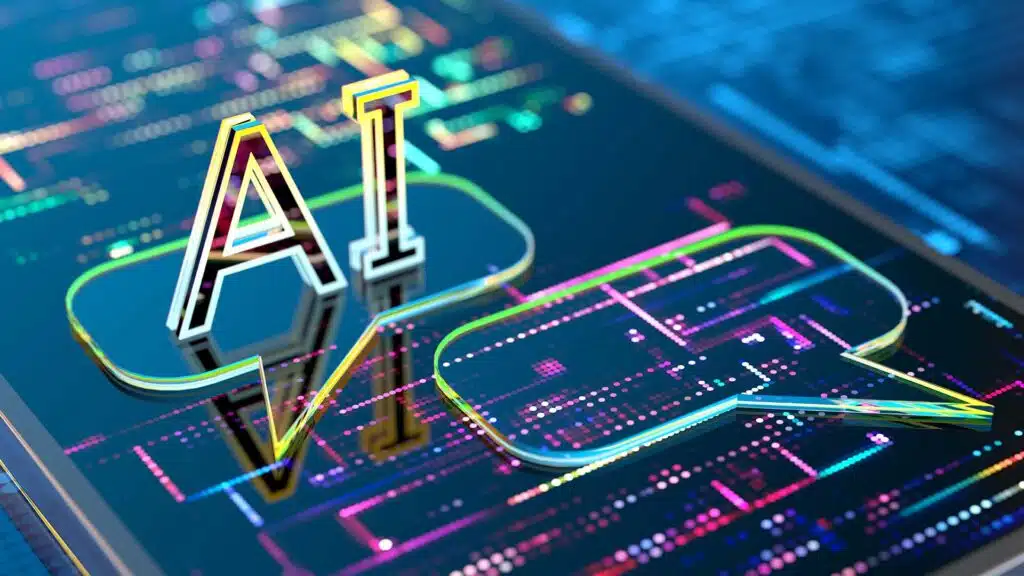
GenAI’s Impact On Hyper-Personalization: Making Every Interaction Count
Explore GenAI's transformative role in hyper-personalization. Elevate customer experiences and drive significant impact now.
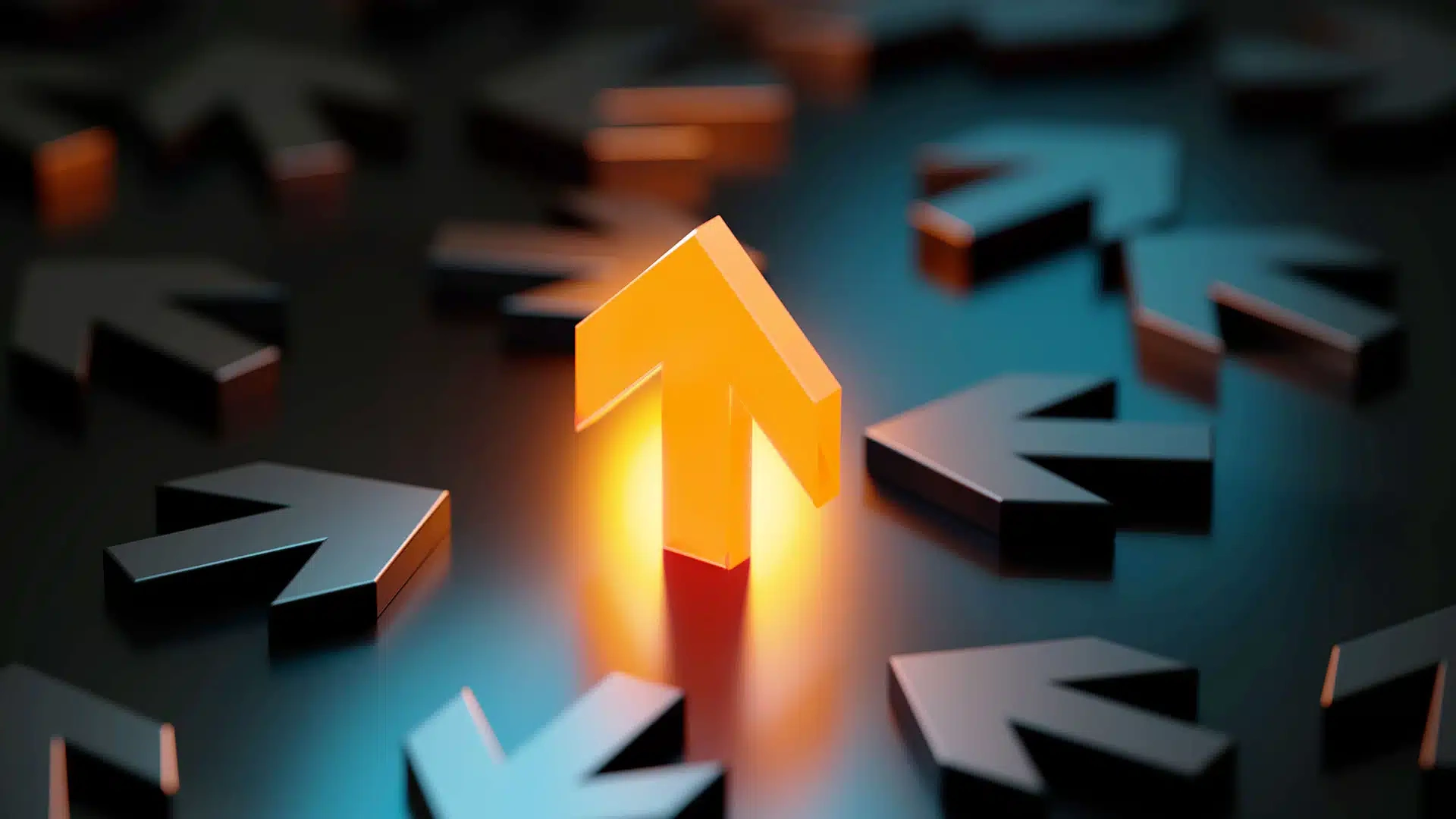
Capital Market Operations Transformation: Why Multiprong Approach is the Need of the Hour
Moving over the traditional approach and embracing a diverse set of methods and tools for effective capital market transformation.