The Big data vs data analytics
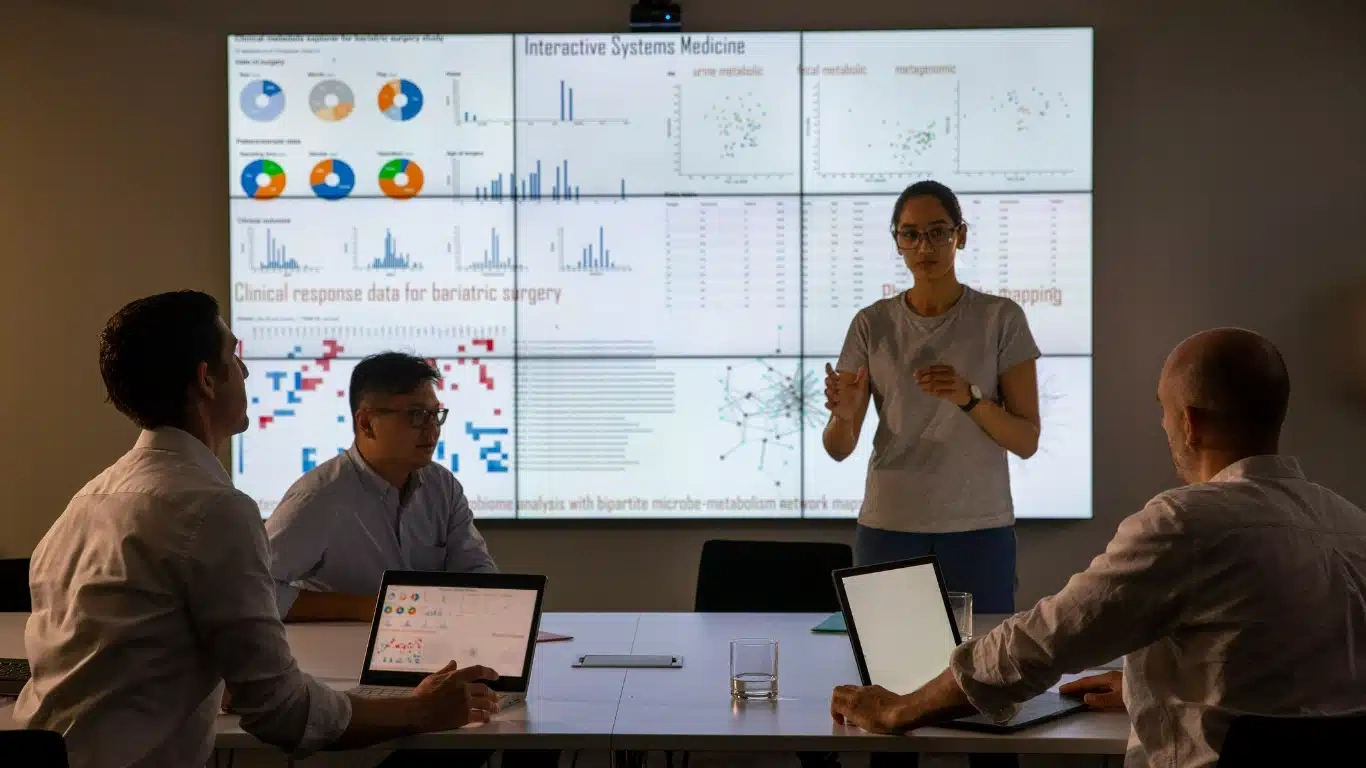
Big Data, Data Analytics – the techie buzzwords are very popular these days but with such a rapid advancement in technology and new concepts coming in every day, the trend is of super-specialization. This leaves us with ideas and technical knowledge which is quite close together, just a little different from one another. Confusion is obvious – for the uninitiated audience, it is tough to find the slight nuances and differences between terms that seem the same. Also, with data almost doubling in volume every two years (which is super-rapid, really) and things getting bigger and bigger each passing day, data technology must keep up as well. Result: newer concepts and systems being employed to manage the huge corpus of data humans are creating, sharing and uploading! Big Data and Data Analytics are two common terms used in the context of information explosion of today but often confused with, used interchangeably when they are not to be replaced with each other. Here are the key differences between the two:
- While Big Data can be compared to a huge and overwhelming library of many books, Data Analytics is one book out of that library where you can find the information.
- Big data is a humungous collection of data – all data, raw and unfiltered and stored in one place. It is generic and the volume of the sheer information it holds can be overwhelming. The format is haphazard, often a mixture of many different formats. Data Analytics revolves around deriving relevant results and information out of the corpus of raw data to solve some problems or show some changes over time.
- Big data uses a lot of complex computational and technological tools (like parallel computing) to source, collect, store and save the amounts of data coming in. Data Analytics platforms uses relatively simpler tools which are predictive and statistical.
- A Big Data professional deals with huge amount of heterogeneous data, which is gathered from various sources coming in at a high velocity. Data analysts translate numbers into a format which can be easily understood. Every business collects data, like sales figures, market research, logistics, or transportation costs. A data analyst’s work is to take that data and use it to help companies to make better business decisions.
- To become a Big Data expert or a professional, one needs the following qualities: A good understanding hold over technologies, the creativity to collect, interpret and analyse a data strategy, knowledge of programming languages like Java, Scala and Frameworks like Apache or Hadoop and good mathematical and statistic skills to help with number crunching.
- For Data Analytics professionals, these qualities are important: Programming languages like R, Python, data wrangling skills to gather raw data and convert it to a presentable format, statistical and mathematical skills to draw conclusions, and very good data visualization skills.
- Big data professionals make data look simple, easy and convenient to manoeuvre through. They restructure the presentation of data so that non-experts can also understand it easily. Data analysts help make the data personalised for a specific person or problem. They come up with ways to make the data arrange in a way that the consumer feels that it has been personalised according to his choices and needs. the data analyst also works in filtering the data the way the consumer might appreciate.
- Big Data consists of the 6 V’s – Volume, Variety, Velocity, Veracity, Value, Variability. The tools with a Data Analyst are R programming, Python, Tableau Public, SAS, RapidMiner, KNIME, QlikView, Splunk.
- Big data affects sectors like Retail, Banking and investment, Fraud detection and analysing Customer-centric applications, Operational analysis etc. Data Analysis Traveling and transportation, Financial analysis, Retail, Research, Energy management and Healthcare.
Data Analytics is very quickly becoming a vital tool for businesses and companies of all sizes. It gives enterprises deep insights into what their customer needs, in today’s era of hyper-personalization, which helps them always stay ahead and relevant. Data-driven businesses are worth $1.2 trillion collectively in 2020, an increase from $333 billion in the year 2015. Similar data highlights the importance of Data Analysis. Thus, in the times when such importance is being given to Data Sciences, we must up our awareness and fall in place with the times. Hence, investing in data analytics services is crucial for businesses aiming to leverage these insights to stay competitive in the market.
Authored by Admin